MND significantly improves the selection of EV charge point locations
- May 17, 2022
- 3 min read
Updated: May 19, 2022
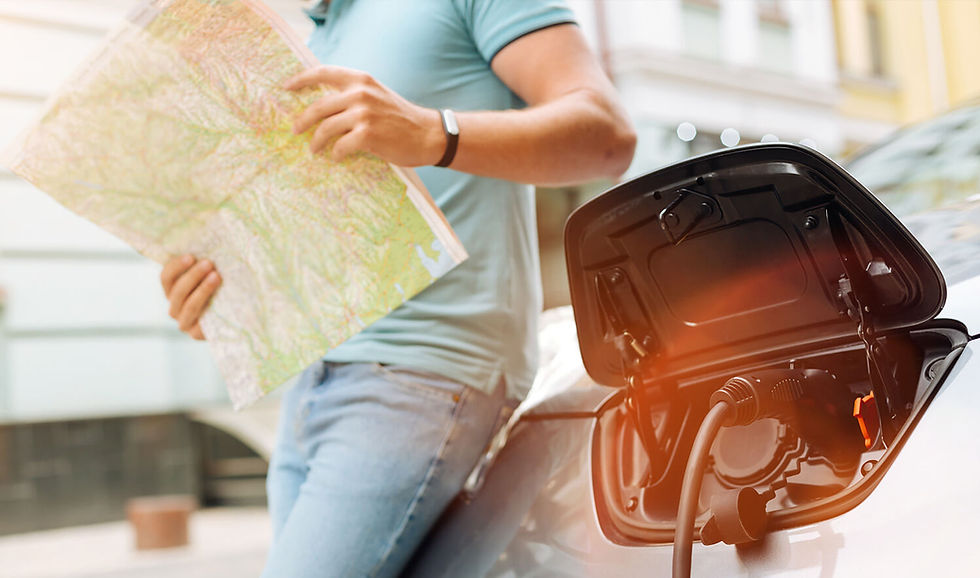
As outlined in a previous post, the rollout of electric vehicle (EV) charge points is lagging behind the adoption of EVs. As more and more drivers are making the switch to EV, it is more important than ever that new sites are installed in optimal locations.
Working with industry partners, Citi Logik found existing charge point sites performed inconsistently. In one example, a site performed four times better than another, despite them having very similar profiles and traffic counts. Charge point operators (CPOs) are understandably keen to identify why such discrepancies occur.
Implementing even a small two-device charge point can cost upwards of £100k, so operators look to ensure sites will make a good return on this investment in the medium-term. This is even more important as EV charging technology is still evolving rapidly, sometimes necessitating expensive upgrades in the short to medium term.
Finding out how MND can help
Given these concerns, Citi Logik started a research project to determine whether mobile network data (MND) could help understand site performance. But before that, the team looked at the traditional and intuitive information sources used by CPOs to see which factors had the biggest impact. This analysis included:
number of competing rapid chargers within 5km
distance to the nearest major junction
time of day & day of week
geographic region
number of chargers at the site
total traffic count around the site
Using a sample of existing chargers provided by Osprey Charging Network, Citi Logik was able to look for a correlation between these various sources and the actual utilisation of the EV chargers.
Old vs new methods
The results surprised even the Citi Logik team. Victor Sequi, lead analyst on the project said; “When we looked at the correlations, we found there was basically no relationship between the variables.”
The team then brought in the power of MND, starting by constructing a model for electric vehicles to improve the results. “When we looked at the EV traffic data as a whole, we found a very weak correlation with the charge point performance figures,” said Victor.
"When we looked at the EV traffic data as a whole, we found a very weak correlation with the charge point performance figures."
Next, the Citi Logik team started to break the traffic down into segments based on a variety of factors such as distance from origin, trip length, dwell time, etc. This led to the creation of hundreds of different trip profiles. “Thanks to MND, we have a huge dataset,” said Victor. “This means we can create lots of segments to increase the granularity, but without losing the quality of the sample size.”
A closer look at the results
Once the segments were tested against the charge point performance data, results improved, doubling the strength of the correlation compared to the homogenous traffic data. These results were encouraging, but the team wanted to go a step further and look for differences in the sites. Rik Muschamp, head of product said; “We figured the sites would likely have different profiles. Some would be used mainly by local customers while others might be see more visitors on long-distance trips.”
“Our proprietary traffic segments correlated so well with retail sites, we were even able to construct a linear model to estimate site performance based solely on the mobile network data.”
This method of splitting the sites into groups – combined with the traffic segments - proved to be a crucial step. One of the groupings – sites in retail locations – exceeded all expectations. “Our proprietary traffic segments correlated so well with retail sites; we were even able to construct a linear model to estimate site performance based solely on the mobile network data.” Victor said.

The strong results extended further. When other site groups were considered – such as restaurants or rural locations – different traffic segments correlated too. These correlations were strong enough to determine threshold values that could eliminate poor sites before they are even installed.
A step towards change
“These results mean we can help operators assess locations for new charge points with much more confidence than the traditional data sources they were using,” said Rik. “Use of our algorithms can have a tangible financial impact on the rollout of new charge points and optimise placement for EV owners.”
Comments